You’re not going to want to miss this! Heard anything of machine learning initiatives conquering almost all industries in the world? Even though it is a set of complex algorithms in the data-driven environment, implementing it in your business can be a real game changer!
As soon as you effectively oversee the complete machine learning lifecycle - from data gathering and model training to deployment and continuing maintenance, you will face the true challenge. Is it something you have to be afraid of? of course not! Otherwise, we would not be even writing this article for you to know how to handle that process. This is a part where we open up all the cards - machine learning operations, or MLOps.
It is about to get real as the global MLOps market size was valued at USD 720.0 million in 2022. Moreover, according to Fortune Business Insights, it is expected to grow from USD 1,064.4 million in 2023 to USD 13,321.8 million by 2030, at a CAGR of 43.5%. You may start wondering why it is so commonly used and popular nowadays. The answer is simple - MLOps offers a comprehensive framework to optimize workflows, improve teamwork, and guarantee the dependability of models in production. It works by fusing the ideas of DevOps with the particular requirements of machine learning projects.
To put it simply, MLOps gives you all the methods and resources you need to get the most out of your machine learning investments, no matter your goals, be it to cut costs, speed time-to-market, or enhance decision-making.
Understanding MLOps
We have already given you the general idea of MLOps. Anyway, let’s explore it a bit deeper.
So, machine learning operations represent a set of practices and tools designed to optimize and manage the entire machine learning lifecycle. You may be questioning the reasons why you should implement MLOps into your business, so we are running ahead and would like to provide you with the main reasons.
#1 MLOps reduces manual effort and accelerates time-to-market;
#2 It provides continuous monitoring and maintenance to make machine learning models more accurate and perform well (even over time);
#3 MLOps enables businesses to scale their machine learning initiatives effectively, even when it comes to handling increased data volumes and user demands;
#4 Having followed all the standards and compliance requirements, MLOps can help businesses manage risks related to model performance and data security.
This is straight-up awesome how integrating machine learning with DevOps principles can bridge the gap between data science and IT operations. You should get ready for this:
-
efficient deployment,
-
smooth and seamless monitoring,
-
easy and enhanced machine learning models in production.
This approach ensures that models not only perform well during development but also remain reliable and scalable when deployed in real-world scenarios. We bet you can’t deny how useful this can be for your organization.
How MLOps Streamlines Business Processes
We have already dived into MLOps. But here we are planning to explain why it is something more than just a set of practices. Of course, we are about to disclose more facts about the benefits of MLOps in the next sections. However, we’d like to emphasize what a transformative solution it is for businesses looking to fully leverage the power of machine learning.
#1 MLOps automation minimizes the manual work needed to transition from model development to deployment.
#2 Such an enhanced and aligned cooperation between data scientists, IT professionals, and other stakeholders reduces the chances of any miscommunication.
#3 MLOps leaves no chance for delays and streamlines processes to speed up development cycles and bring solutions to market faster.
#4 All the technical complexities are handled by MLOps, while teams can concentrate on higher-level strategic goals rather than day-to-day operational tasks.
#5 The final (and our favorite) is that MLOps enables machine learning initiatives to grow alongside your business.
In other words, buckle up to shift your focus from the technical aspects of your platforms to striving and chasing more strategic goals. The result? More robust, scalable machine learning initiatives that drive real business value.
DevOps vs. MLOps: Key Differences
It may have you scratching your head as DevOps and MLOps share a common goal of enhancing collaboration and efficiency. Nevertheless, there are some distinct and different purposes they serve. Ready to find them out? Let’s go!
#1 DevOps has the main goal - to optimize software development and deployment processes. It mainly focuses on integrating development and operations teams to improve software delivery.
#2 MLOps, on the other hand, is crafted to address the unique challenges of machine learning. It includes data management, model architecture and validation, continuous monitoring, model deployment, model versioning control, infrastructure management, automation of pipelines, and collaboration across teams.
We hope that it has become more clear to you what the differences are and that you’re following the aspects we have mentioned. Remember that understanding these differences is crucial for organizations looking to implement the right practices for their specific needs.
Defining the Benefits of MLOps
We have already covered the benefits of MLOps briefly earlier in this article. In this section, you will have a great journey of discovering all the nooks and crannies of implementing this top-notch solution.
Accelerated time to market
The ability of MLOps to significantly shorten the time required to develop a machine learning model from conception to production is one of its most important benefits. You should be aware that MLOps enables companies to adjust swiftly to market demands and maintain an advantage over rivals by automating crucial processes like data preparation, model training, and deployment.
Cost reduction
One of MLOps' greatest benefits for businesses is that it optimizes resource usage and lowers the need for manual intervention, which results in cost savings. Automation helps control risk management and lowers the possibility of mistakes that result in expensive rework. Constant monitoring makes sure the models run well and saves money on computational power and cloud resources.
Increased model reliability and performance
Be aware that models with MLOps are updated and monitored often to guarantee their continued good performance. This ongoing monitoring makes it possible to spot potential issues like data drift or model deterioration and take prompt action to preserve high standards of accuracy and dependability.
Real-time data insights
This advantage is extremely important since MLOps gives companies the ability to obtain real-time insights from their machine learning systems. Businesses can improve their responsiveness to new trends, customer experiences, and challenges by integrating real-time data pipelines and making decisions based on up-to-date information.
Enhanced decision-making
Is anything better than helping us drive the efficiency of our business decisions? Decision-making procedures are directly impacted by the capacity to produce actionable insights. MLOps gives organizations a dependable framework for implementing models that produce useful intelligence, enabling them to swiftly and confidently make decisions based on data.
Automated monitoring and maintenance
You’re in for a treat! MLOps eliminates the need for continual human supervision by automating the maintenance, monitoring, and management of machine learning models. By automating the process, models are kept current and perform at their best, freeing up time and resources that could be used for other important strategic projects.
Effective risk management and compliance
In order to effectively manage the risks connected to machine learning models, MLOps is essential. MLOps assists companies in reducing risks associated with model bias, data privacy, and security issues by standardizing procedures and guaranteeing adherence to industry standards. This is a genuine solution that is regarded as groundbreaking in the modern era.
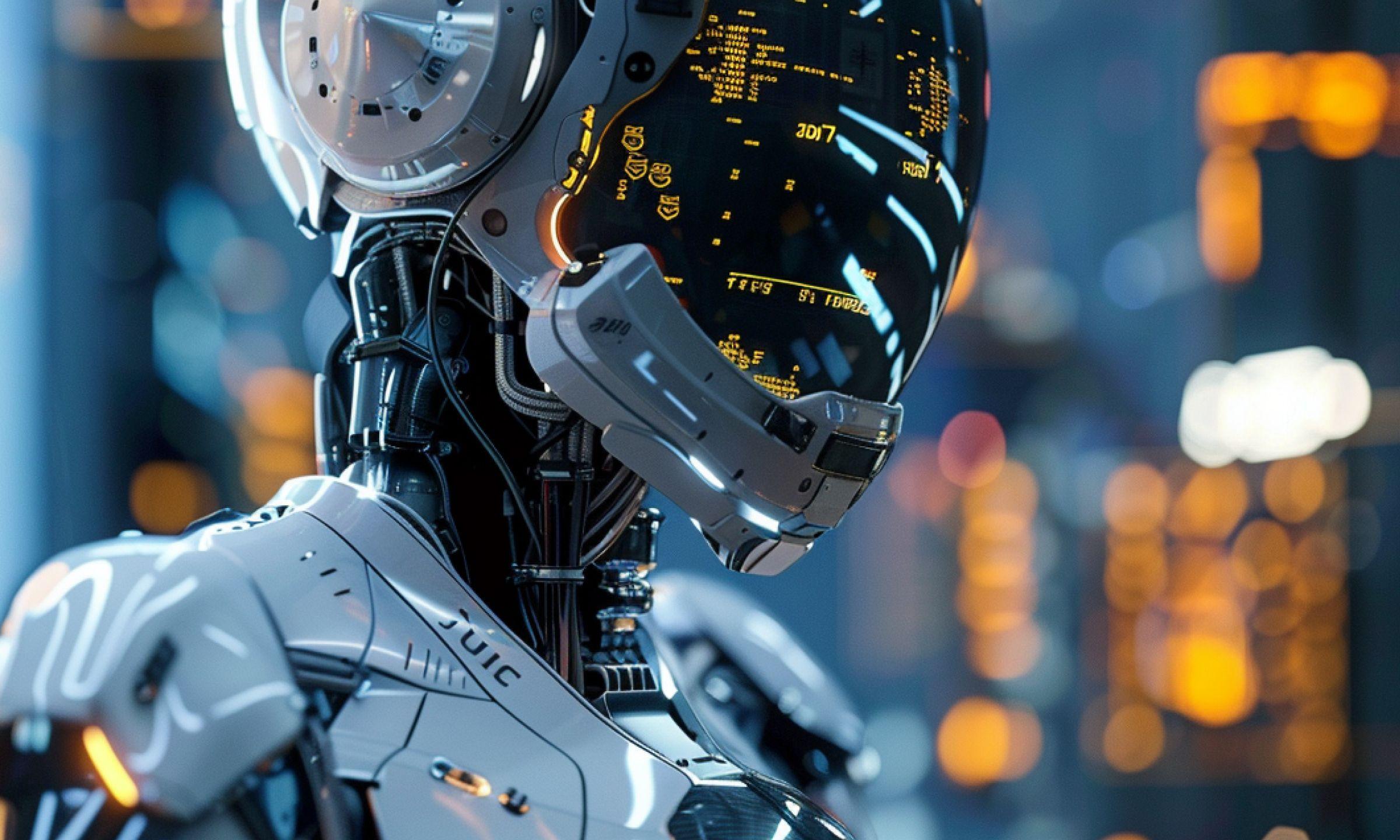
Ready to Enhance Your ML Processes with MLOps?
Contact usCore Features of MLOps Solutions
There is no denying how efficient machine learning operations are. And to make the most of it, we would like to give you more specifics on the core features of MLOps solutions to really elevate your business.
Comprehensive automation
Never forget that the foundation of MLOps solutions is end-to-end automation. Through the automation of processes like data preprocessing, model training, and deployment, MLOps reduces human work and speeds up the entire machine learning lifecycle. Additionally, improving consistency and lowering the possibility of errors are the outcomes of these automation processes.
Scalability enhancement
People will always be concerned about scalability because they don't want to invest in another project after a few years. The scalability of these AI-driven solutions is maximized, guaranteeing that machine learning models can manage growing data volumes and user demands without sacrificing performance. Businesses hoping to grow their machine-learning initiatives will be able to scale this.
Process standardization
It should come as no surprise that standardization is a fundamental component of MLOps, as it offers a uniform framework for creating, implementing, and managing machine learning models. By ensuring that everyone in the team adheres to best practices, this standardization produces results that are more dependable and effective.
Seamless integration with DevOps and CI/CD
An actual marvel of technology! With current DevOps and Continuous Integration/Continuous Deployment (CI/CD) pipelines, MLOps integrates easily. Because of this integration, machine learning models are created and implemented with the same imperative standards as traditional software, which results in models that are more durable.
Democratizing machine learning
By making machine learning available to a wider range of users within an organization, MLOps democratizes the field. MLOps promotes creativity and cooperation by enabling even non-experts to participate in machine learning projects by automating difficult tasks and offering user-friendly tools. It is among MLOps' greatest and most inclusive features.
What are the Best Examples of MLOps Practices?
When you were reading the sections about the key differences between DevOps and MLOps, you might have briefly got acquainted with the main practices machine learning operations represent.
Rigorous code quality checks
Maintaining the dependability of machine learning models requires high code quality. Regular code reviews and automated testing, in our opinion, aid in finding and fixing problems early in the development process, creating models that are more reliable and manageable.
Thorough data validation
Data validation is, in our humble opinion, an essential step in the MLOps pipeline. Organizations can guarantee that their models are based on correct and pertinent data by carefully validating data before using it for training. This lowers the risk of errors and improves overall model performance.
Model validation and testing
Model validation is a continuous process in MLOps and you should be ready for that. It involves rigorous testing (like unit tests, integration tests, etc.) to ensure that models perform well in various scenarios. By identifying potential flaws and enabling prompt adjustments, this testing ensures that models continue to function well in practical applications.
Collaborative work environment
Bear in mind that collaboration is key to successful MLOps implementation. Organizations can make sure that all stakeholders are on the same page and pursuing the same objectives by cultivating a culture of cooperation and communication. Better results and more efficient automated processes are the results of this collaboration.
Encourage experimentation
Isn't experimentation at the core of machine learning innovation? Teams are encouraged by MLOps to experiment with various models, algorithms, and techniques in order to gain fresh insights and make ongoing improvements to their models.
Continuous monitoring
Not to mention, ongoing monitoring is an essential part of MLOps. It offers a live view of the model's performance. Through early detection and resolution of problems, this monitoring enables organizations to maintain the accuracy and dependability of their models over time.
Who Benefits from Implementing MLOps?
Will you be surprised if we tell you that MLOps is beneficial to a wide range of stakeholders within an organization? Exactly! So, who and how can feel all the advantages of using MLOps?
#1 Who - Data Scientists. How - streamlined processes (it is much easier and more efficient when it comes to developing and deploying machine learning models) and reduced manual tasks (automated workflow and minimized errors).
#2 Who - IT Teams. How - reduced complexity (it is way easier to manage machine learning infrastructure) and smoother integration.
#3 Who - Business Leaders. How - faster time-to-market and reliable insights (it brings access to accurate data for better strategic decision-making and innovation).
#4 Who - Organizations. How - effective use of machine learning across departments and improved outcomes.
Elevate Your Business With Our MLOps Services
The technological environments are developing so rapidly that it may be getting more and more challenging to stay ahead of the competition. However, our dedicated team at OTAKOYI knows what is required to properly approach managing the entire machine learning lifecycle.
The moment you partner up with OTAKOYI for MLOps services, you get a chance to enjoy several key advantages.
#1 Cutting-edge solutions
You can benefit from the latest advancements in technologies to keep your machine learning initiatives at the forefront of technology.
#2 Reliability
We ensure that your models are robust and dependable, with continuous monitoring and maintenance.
#3 Scalability
The OTAKOYI team can seamlessly scale your machine-learning solutions to handle increasing data volumes and user demands.
#4 Alignment with business goals
Our developers, engineers, business analysts, and managers are eager to align your MLOps strategy with your specific business objectives to drive only meaningful results.
#5 Expert support
You can leverage our expertise to navigate the complexities of the machine-learning lifecycle effectively and smoothly.
Let OTAKOYI help you unlock the full potential of MLOps and drive your business forward with ease and confidence!
Wrapping Up
To cut a long story short, MLOps is a next-level need for all modern businesses looking for ways to efficiently automate their process and make them serve them well. Artificial intelligence has already turned the processes of decision-making upside down. So, make sure to cooperate with experienced and dedicated engineers to choose the best MLOps practice for you. Don't hold up! The machine learning market is gaining traction with every coffee break you take. See you at OTAKOYI!