Do you know anyone who still hasn’t heard of AI? Everyone involved in the business world is directly or indirectly concerned with AI development. It’s getting more and more traction, both simplifying and replacing jobs. How do you make these solutions serve you well? If you are looking for the answer to this question, you’ve reached the right address. First things first, let us mention that we’re about to disclose the differences between NLP vs. LLM. Have you heard that the global LLM market is expected to grow from $1,590 million in 2023 to $259,8 million in 2030? Moreover, the CAGR will reach 79,80% during 2023-2030. At the same time, the NLP market overall is anticipated to reach an impressive value of USD 453.3 billion by 2032. Sounds exciting, right?
Let’s break it down: NLP and LLMs are the real deal in the world of AI, but they aren’t the same thing. Each approach has its perks, and together, they’re changing how we handle language and data. That’s what we are about to find out.
Unpacking the Basics of Natural Language Processing (NLP)
To put it simply, NLP is like AI’s language expert. It helps computers get what we’re saying, whether it's chat messages or analyzing documents. It’s all about making sense of human language in a structured way—no fluff, just the facts. To break it down into bucket list, NLP:
-
can get the main information from massive texts and databases;
-
chats and, in general, interacts with people;
-
accurately translates various languages;
-
can even analyze opinions and emotions.
What is the comparison of NLP vs. LLM? First, let’s find out what LLM is!
The Essentials of Large Language Models (LLMs)
Large language models (LLMs) are also types of deep learning algorithms capable of handling a wide range of natural language processing (NLP) tasks. LLMs are the big brains behind all AI conversations. Sounds exciting, right? They take language to the next level, generating human-like language responses and creating content. Think of them as your go-to for more creative and free-flowing text. The key points of LLM capabilities:
-
it can generate human and high-quality text (if you don’t know how to write poems but you’d like to impress your loved ones - you know what to do!);
-
it can differentiate complex contexts and has a natural language understanding
-
LLM can tailor messages and act as a human (without prior programming, so to speak).
As you can see, these solutions could work perfectly well together. But what makes NLP and LLM different?
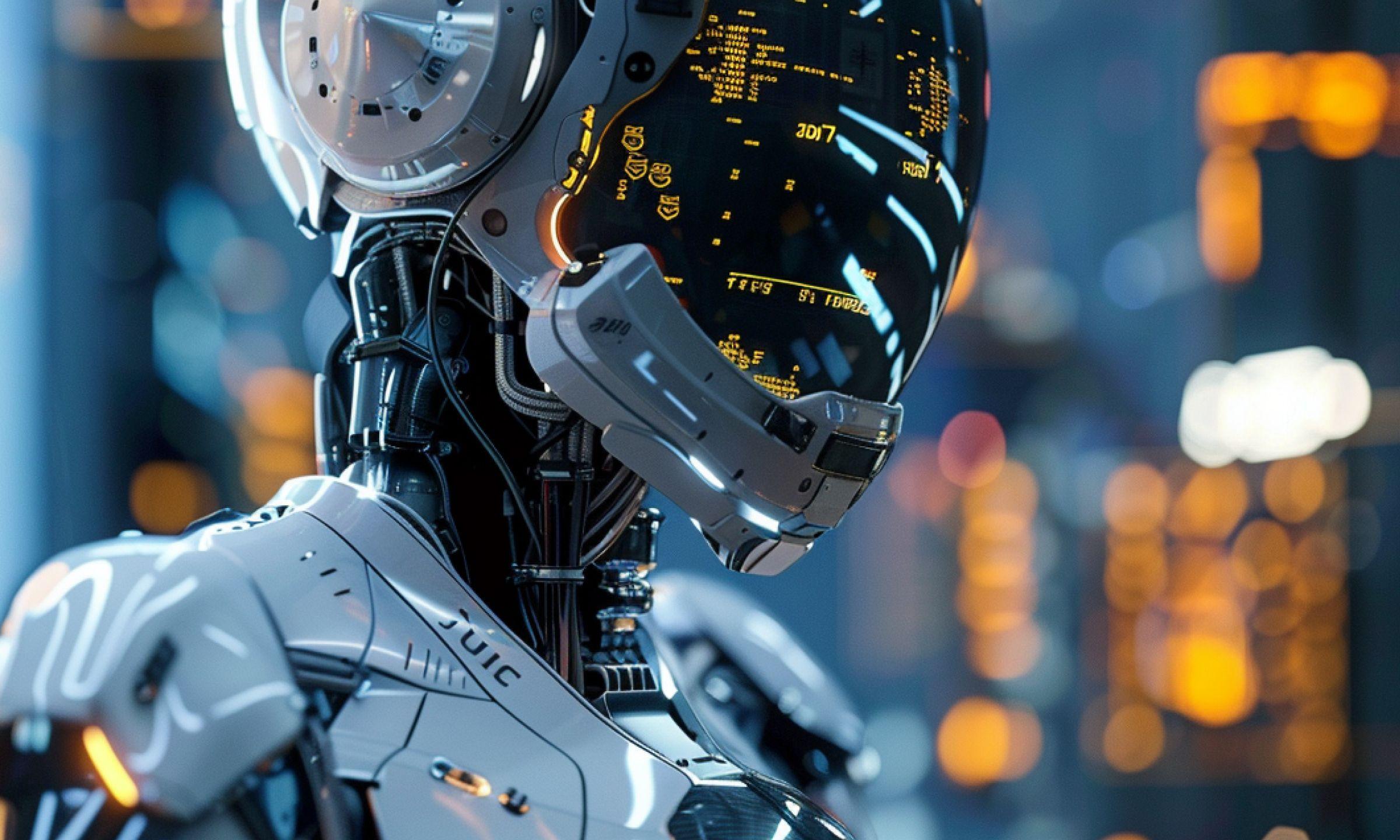
Discover how NLP and LLM solutions can enhance your operations
Contact usTraditional NLP and LLM: What Sets Them Apart?
And here we are! So now we are going to find out the differences NLP vs. LLMs represent. While reading the differences, you’ll also discover their core advantages to make it easier for you to make more informed decisions.
So, even though traditional NLP and LLMs are both AI-powered, they each have their own lane. Let’s see where they shine and where they struggle.
Scope and real-world applications
Defining the difference between them, we are going to mark it this way.
Traditional, often also called classic, NLP is the go-to for handling structured tasks like customer support or analyzing reviews. On the other hand, LLMs are all about creativity, content generation, and having real conversations.
Traditional NLP vs. LLM – different tools for different jobs.
Levels of technological complexity
Traditional NLP models are usually simple, like decision trees or linear regression. Usually, it is used for more straightforward tasks. It's effective for structured, rule-based processing. At the same time, LLM leverages advanced deep learning architectures. It requires substantial computational resources and large datasets. Unlike simpler NLP models, it's designed for tasks that demand a nuanced and deep understanding of language.
As you can see, NLP vs. LLM – both get the job done, just in different ways.
Resource demands and efficiency
As we have already mentioned, classical NLP models typically requires smaller, task-specific datasets and fewer computational resources. There are many pre-trained NLP models, which make it easier to fine-tune them using transfer learning. It is efficient for simple tasks like topic modeling, entity extraction, or modeling of language. It also uses minimal resources.
When speaking about LLM, it demands vast datasets and immense computational power and capacity. There are billions of parameters, so training LLMs is costly and resource-intensive. Even though you get more sophisticated and in-depth results, you should be ready for much higher resource expenses.
Task performance in language processing
Classic NLP is applied to well-defined, structured tasks (like named entity recognition, sentiment analysis, or text classification). Nevertheless, Natural Language Processing capability is highly efficient and accurate.
LLM, on the other hand, is required when tasks involve complex language understanding, generating human-like responses, or processing complex, ambiguous, and unstructured data. Most often, LLMs outperform NLP models in this matter. With their deep learning capabilities, LLMs can handle intricate language tasks, such as open-ended conversations, creative content generation, or a deeper understanding of nuanced context.
Overall, classical NLP vs. LLM serve a bit different purposes, but perform them for 100%!
Ethical and legal implications
We have reached the most interesting part - what legal and ethical implications do traditional NLP and LLM stumble upon?
We would like to start with LLMs. Due to their large-scale language model and ability to generate highly complex outputs, LLMs pose significant ethical and legal concerns. They can unintentionally spread biases embedded in their extensive training data. Result? It can lead to problematic or discriminatory outputs. Moreover, LLMs can be misused for malicious purposes (we’ve all observed deepfakes and misinformation spreading on the internet, right?). Consequently, these advanced models also raise privacy concerns. That is why it is important to collaborate with experienced engineers who know how to address these issues and prevent misuse.
Traditional NLP models, in comparison with LLMs, possess a much smaller scale of concerns (it is because of not such a wide range of scope and simpler structure). However, NLP models are widely deployed in various industries. That is why even minor biases can have a broad impact. What are the risks? It may affect automated hiring processes, customer service, or even legal document processing.
Therefore, ethical scrutiny and fairness in data training of both NLP and LLM are crucial and require a rigorous approach.
Challenges and limitations
Even though both classical NLP and LLM are advanced and always-progressing solutions, there are still some obstacles they face.
NLP, for example, can be limited by strict rules and misread some texts. The challenge is to make it understand everything in the right way. The example can be a solution that can tell us which text is AI generated. It is a very speculative topic, right? Many solutions can show different results, which misleads users.
Meanwhile, LLMs sometimes overreach and generate answers that might sound convincing but miss the mark. One more challenge is ethical consideration - how far LLM can reach in mimicking human communication? Something that needs a second thought.
Both NLP and LLM have their challenges, but they’re always improving.
LLM and NLP: Various Use Cases of the Technologies
NLP and LLM applications are expanding along with the development of AI. Their applications have grown more diverse in the last few years. The list of industries that implement these technologies is expanding, as well as use cases. We would like to get you acquainted with the most common and efficient ones. So, let’s discover the practical applications, and maybe you’ll find something for yourself.
1 Customer support automation (NLP & LLMs)
-
Chatbots and virtual assistants
-
Sentiment analysis
2 Content creation and summarization (LLMs)
-
Automated article writing
-
Text summarization
3 Machine translation (NLP & LLMs)
-
Real-time language translation
4 Healthcare industry (NLP & LLMs)
-
Medical record analysis
-
Drug discovery
5 Voice assistants (NLP & LLMs)
-
Voice recognition
6 Legal tech (NLP & LLMs)
-
Document review and compliance
7 Fraud detection (NLP)
-
Financial fraud prevention
8 Education (NLP & LLMs)
-
Language learning
-
Essay grading and feedback
9 Market research and trend analysis (NLP)
-
Consumer behavior insights
People say that the best way to discover how NLP and LLM work is to see the real solutions. That is why we invite you to discover the OTAKOYI solutions like AI Digital Platform for Vehicle Search, Legal Mates, and others to see how the magic works!
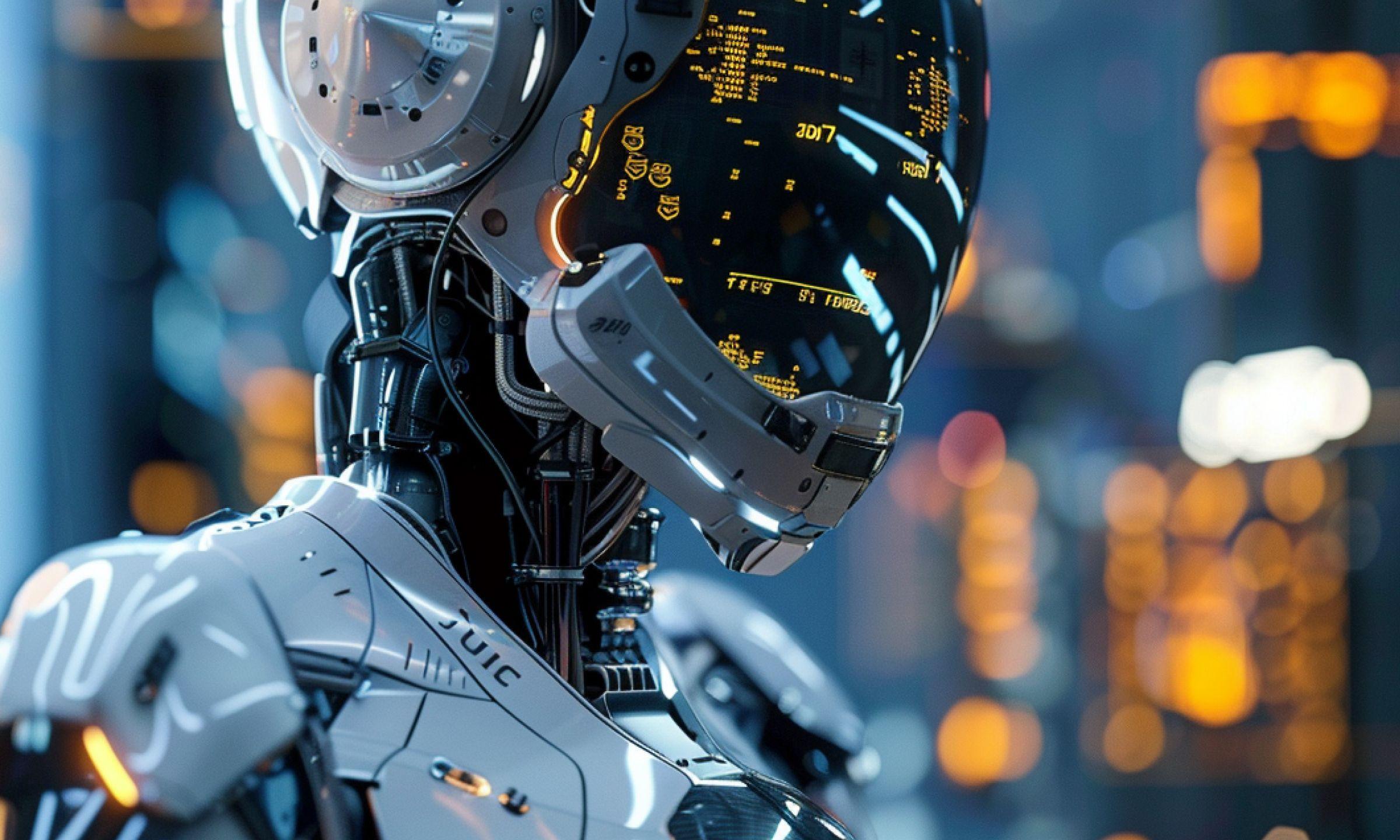
Have you found the perfect use case for your business?
Contact usFuture Outlook: Will NLP and LLMs Merge?
The future looks promising for the integration of multiple NLP and LLMs. A lot of experts do expect this combination to unlock new possibilities for businesses and tech. As these solutions are evolving, they are simultaneously revolutionizing how we communicate with AI. As a result, such interactions are becoming more and more intuitive and human-like. That’s why the merge of both is possible and game-changing.
#1 Smarter AI assistants
One can basically merge LLM’s creativity and NLP’s precision. This way, AI assistants can understand not only what we say but also the text-based content and emotion behind it. It may give goosebumps, but this means they might respond more naturally. ready for AI interlocutors?
#2 Revolutionized content creation
As soon as you combine classic NLP and LLM, start imagining tools that can write blog posts, summarize reports, and even create art with ease. Traditional NLP is to take responsibility for the structure and rules, and LLM – for driving creativity. Their automated content creation will meet the needs of diverse industries.
#3 Improved human-robot communication
Looking for more meaningful and smoother interactions? Well, good news! Merging these technologies, robots may soon understand not only commands but also intentions. Humans and machines might reach a new level of communication and collaboration.
Expert Insights
Mykhailo Sokolov, AI Engineer:
LLMs are game-changers when it comes to generating content. Their ability to create human-like responses and summaries creates many opportunities for businesses. For example, to automate creative writing tasks like content writing and personalized customer interactions, which, in turn, significantly boosts efficiency and customer satisfaction.
Mariia Kokshaikyna, Machine Learning Engineer:
NLP is all about understanding structure and meaning. In industries like finance, banking, or legal tech, NLP is especially invaluable for getting key insights from massive amounts of text. It’s also highly effective for tasks like document analysis and compliance. If you have noticed, it really matters for industries where accuracy is crucial.
Experts agree that the real power comes from combining NLP and LLMs. Data scientists highlight how NLP excels at structured, rule-based tasks, while LLMs bring flexibility and creativity. Together, they offer a balanced approach that suits a variety of business needs. However, solutions architects caution that businesses should be mindful of the resource demands of LLMs, as these models require significant computational power. When fine-tuned for specific use cases, though, the return on investment can be impressive.
Wrapping up
As you can see after reading this article, both traditional NLP and LLMs have their unique strengths. Classical NLP shines with its precision and efficiency (e.g., in tasks like customer service, data analysis, and structured tasks). LLMs, on the other hand, bring a creative flair to content creation, advanced language generation capability and more dynamic interaction between computers. The right choice really depends on what your business needs – whether it’s streamlined automation or sophisticated language comprehension tasks.
For many companies, the magic happens when these technologies come together. Combining traditional NLP’s accuracy with LLM’s creative power can unlock endless possibilities for smarter, more intuitive Artificial Intelligence solutions. To make sure you’ve made the right decision, partner up with experienced developers like OTAKOYI and enjoy all the advantages NLP and LLM can bring!